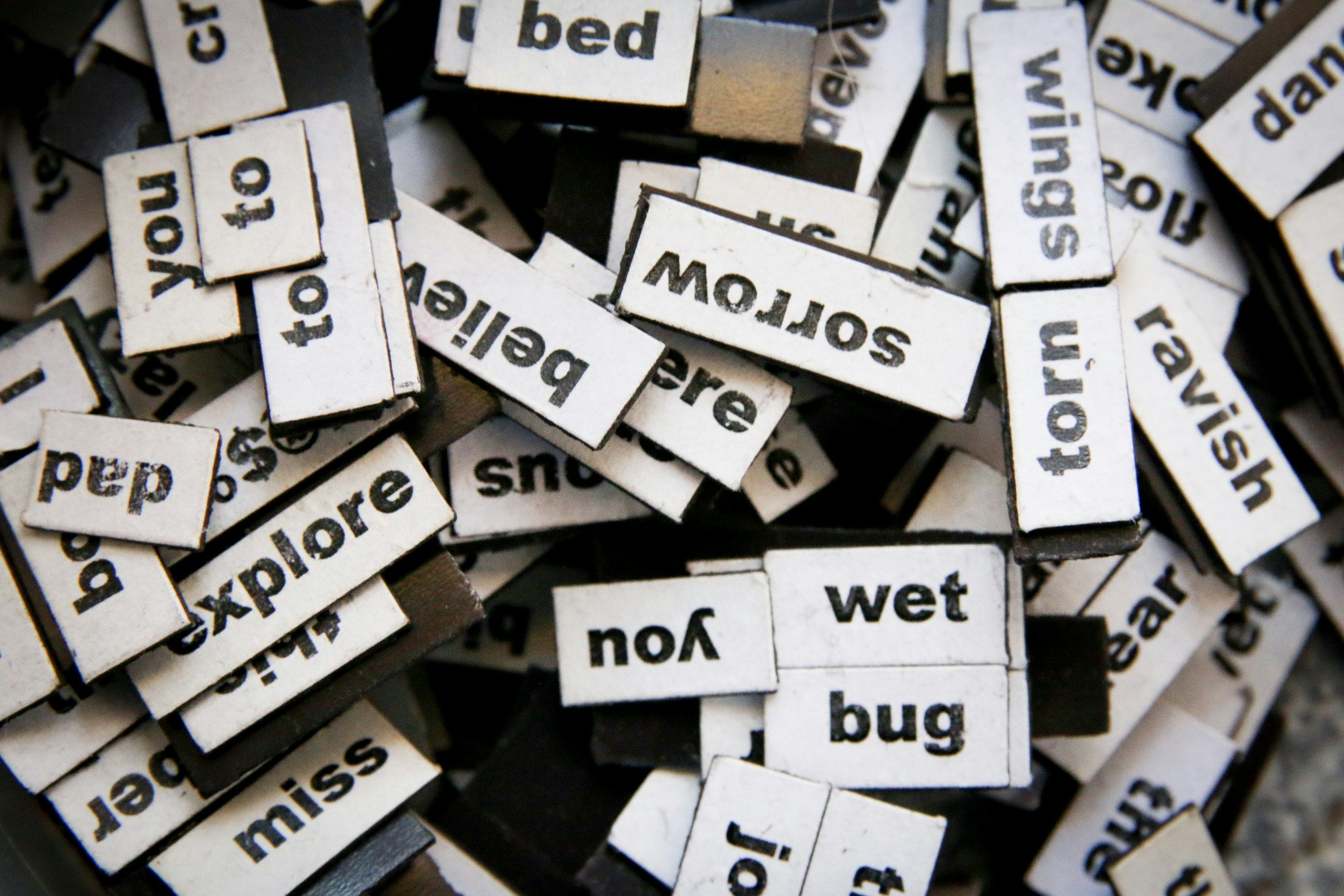
From RAGs to Riches: Multimodal Retrieval
While large language models (LLMs) have made remarkable strides in processing and generating text, they often struggle with visual information. This limitation has led to a text-only bias in many AI systems, where they struggle to incorporate or generate visual content effectively.
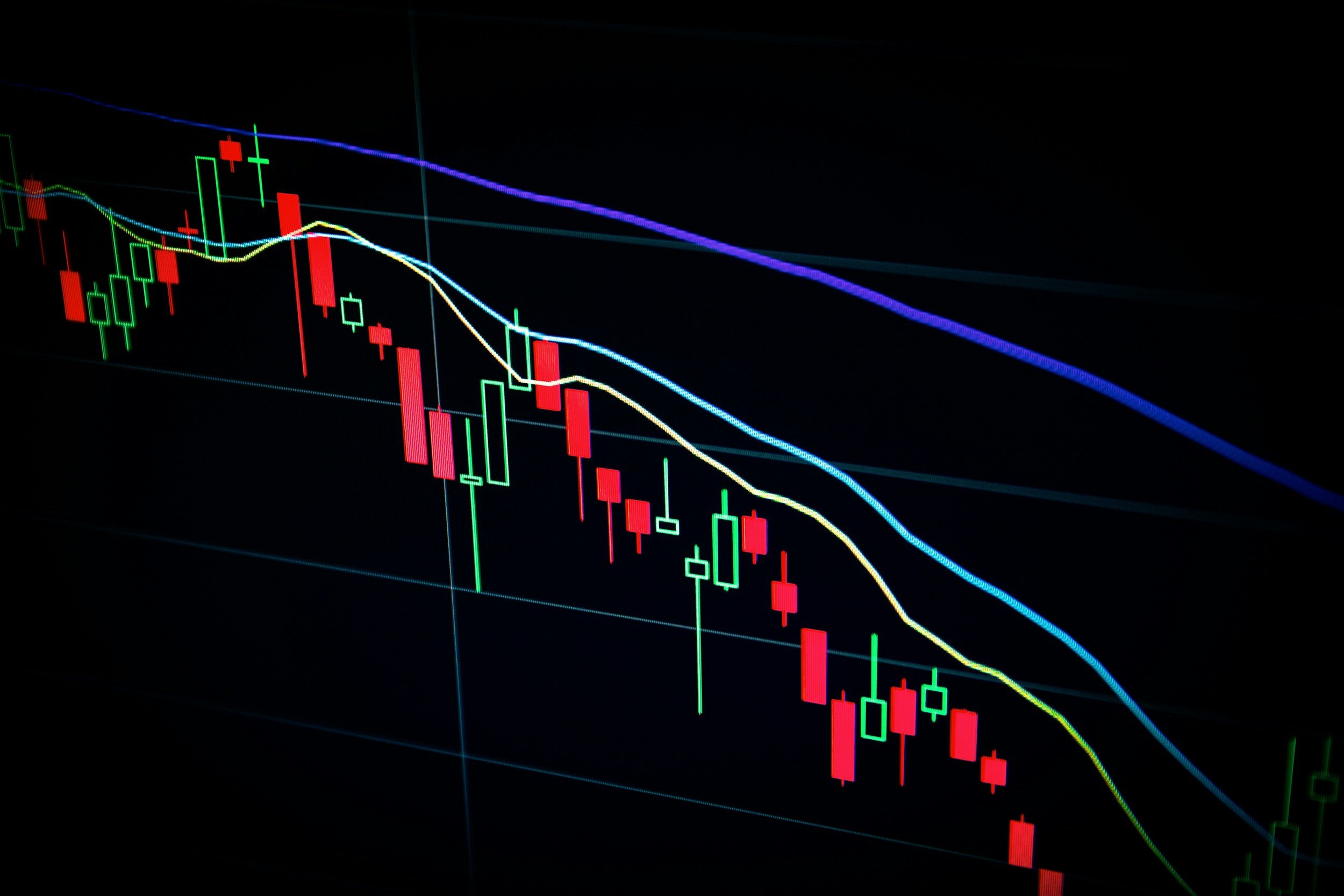
From RAGs to Riches: Real-time Retrieval
Staying up-to-date with real-time events and trends is essential for businesses to make timely decisions and maintain a competitive edge. RAG offers advanced methods for event-enhanced retrieval and generative event argument extraction.
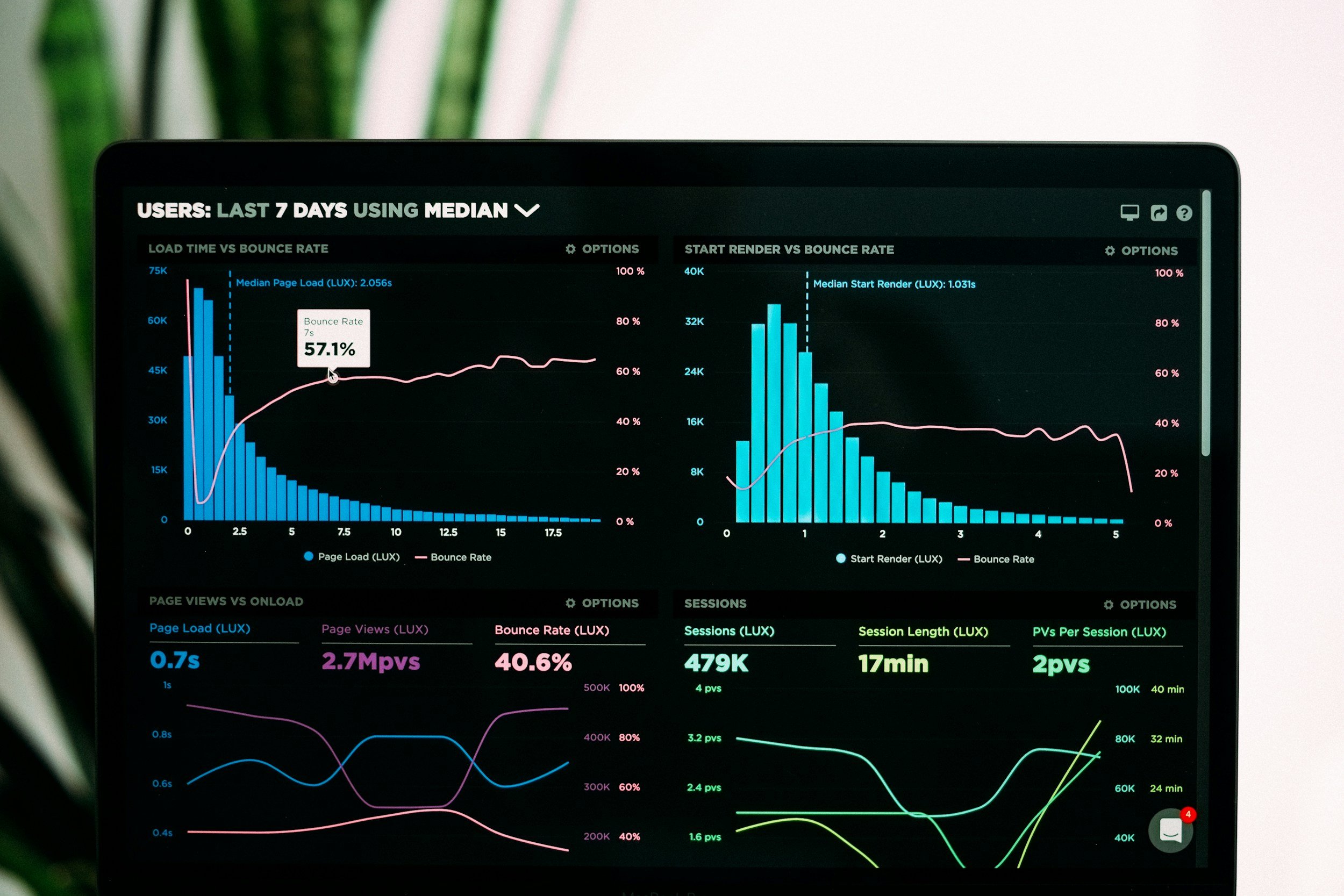
From RAGs to Riches: Data Conflicts
One of the main challenges in RAG is dealing with knowledge conflicts between the pre-trained language model and the external knowledge sources. These conflicts can arise when the information in the external sources contradicts or differs from the knowledge learned by the language model during pre-training. To address this issue, researchers have developed techniques for assessing conflicts and calibrating model confidence.
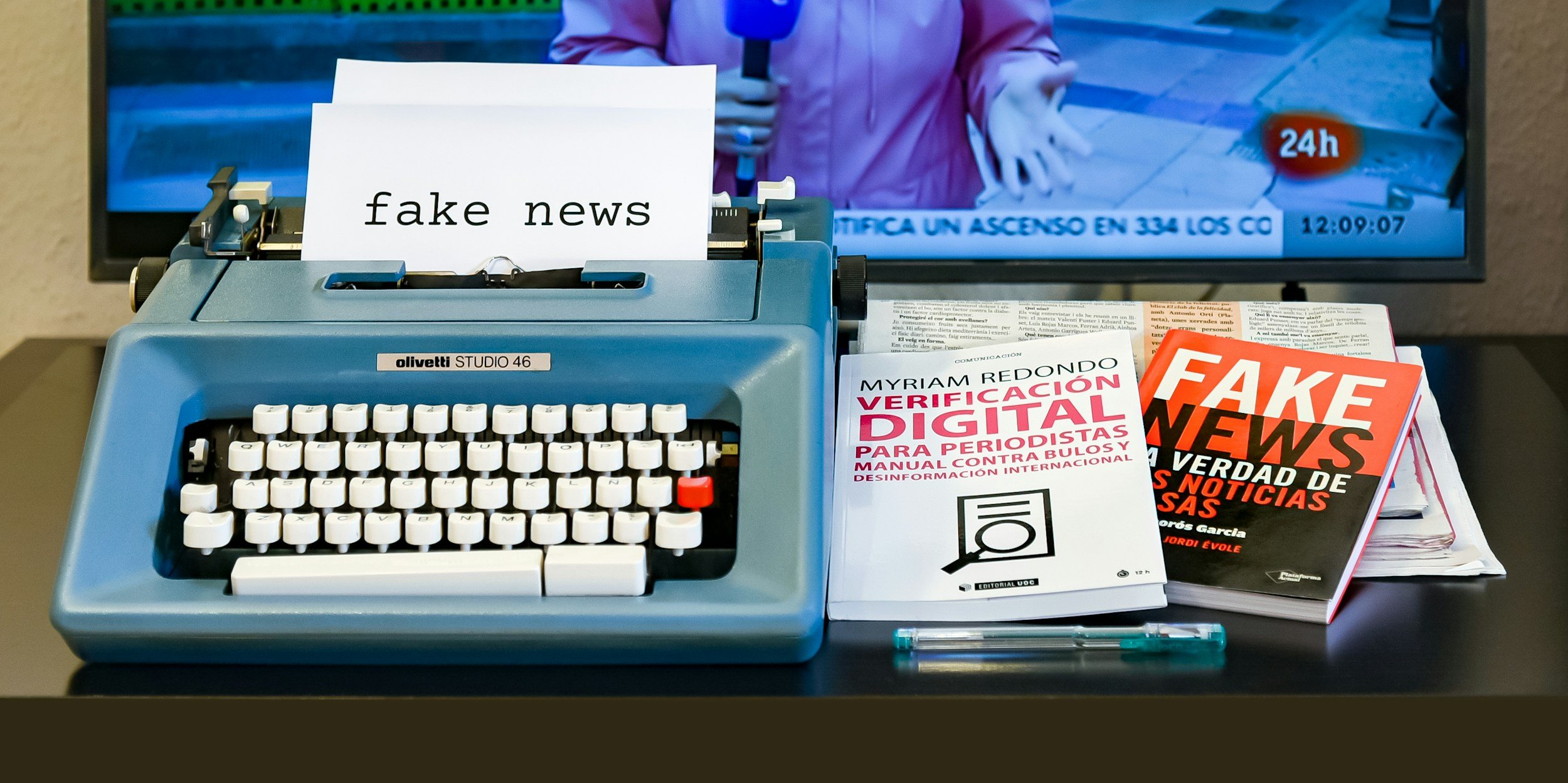
From RAGs to Riches: Misinformation
Imagine an AI assistant that not only understands natural language but also has instant access to the most up-to-date information from your company's databases and beyond. By retrieving relevant information from external sources and integrating it with the LLM's output, Retrieval Augmented Generation (RAG) ensures that generated text is not only fluent but also accurate and applicable to the user's specific needs.
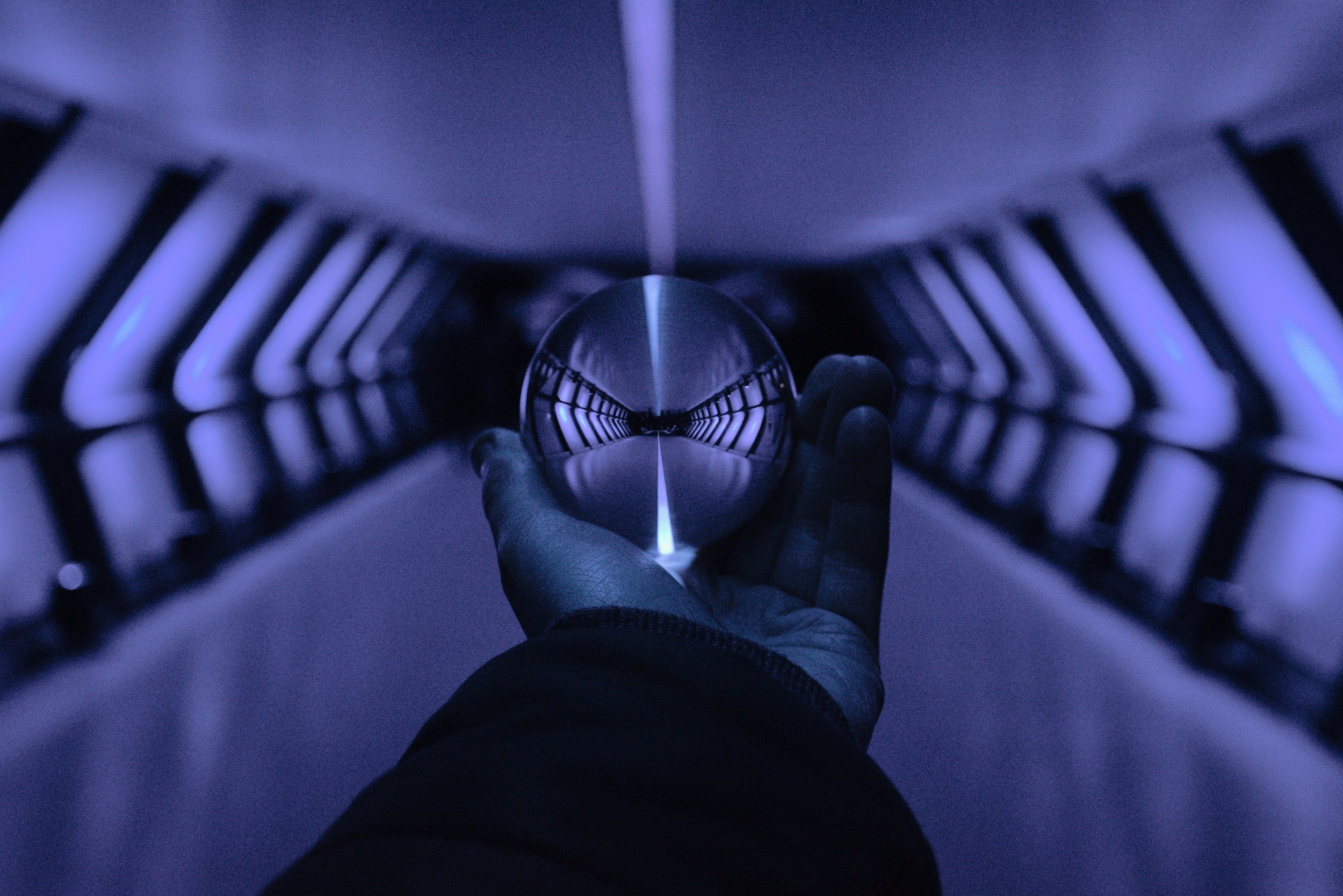